Adversarial Machine Learning: AI and ML Beware
2024-09-30 Terry Gaul
Artificial Intelligence (AI) and machine learning (ML) technologies are on an accelerated trajectory, finding their way globally into mainstream systems, devices, and critical applications as governments, commercial, and industrial organizations grow increasingly connected. Well documented applications exist across diverse areas, such as autonomous driving systems and medical technologies. However, much like cybersecurity risks inherent in IoT devices and IIoT systems, AI and ML technologies are similarly vulnerable to attacks that can cause dramatic failures and catastrophic consequences.
According to the U.S National Institute of Standards and Technology (NIST), “for all its potentially transformational benefits, generative AI also brings risks that are significantly different from those we see with traditional software.” In January 2024, NIST published details about a type of cyberattack unique to AI systems: adversarial machine learning where attackers can “corrupt” or “poison” data that might be used by AI systems for training, thereby causing those AI systems to malfunction.
Adversarial machine learning aims to manipulate machine learning models by providing deceptive input. These deceiving inputs can cause a machine learning model to malfunction, potentially exposing data or disrupting the function operated by machine learning.
A simple example used in a study conducted by researchers from Princeton, UC Berkely, and Purdue, underlined the potential danger involved in adversarial machine learning on the manipulation of autonomous vehicles. Self-driving vehicles use machine learning models to interpret road signs. Slight modifications to these street signs, such as the placement of a sticker on a yield sign, can cause the machine learning model to malfunction.
The NIST report outlines four major types of attacks: evasion, poisoning, privacy and abuse attacks. It also classifies them according to multiple criteria such as the attacker’s goals and objectives, capabilities, and knowledge.
- Evasion attacks occur after an AI system is deployed and the attacker attempts to alter an input to change how the system responds to it. As mentioned earlier, examples would include adding markings to stop signs to make an autonomous vehicle misinterpret them as speed limit signs or creating confusing lane markings to make the vehicle veer off the road.
- Poisoning attacks occur in the training phase by introducing corrupted data e.g., slipping numerous instances of inappropriate language into conversation records, so that a chatbot interprets these instances as common enough parlance to use in its own customer interactions.
- Privacy attacks occur during deployment and attempts to learn sensitive information about the AI or the data it was trained on in order to misuse it. An adversary can ask a chatbot numerous legitimate questions, and then use the answers to reverse engineer the model so as to find its weak spots — or guess at its sources. Adding undesired examples to those online sources could make the AI behave inappropriately, and making the AI unlearn those specific undesired examples after the fact can be difficult.
- Abuse attacks involve the insertion of incorrect information into a source, such as a webpage or online document, that an AI then absorbs. Unlike the aforementioned poisoning attacks, abuse attacks attempt to give the AI incorrect pieces of information from a legitimate but compromised source to repurpose the AI system’s intended use.
These types of attacks are most likely just the beginning. No doubt, as AI and machine learning use cases increase, so will the type and scale of attacks on the data.
As a company dedicated to IP protection and data security, safeguarding AI and ML data is high on our list of priorities. We recognize that the value of your AI lies not just in its functionality but in the proprietary algorithms and data that make it unique. In addition to protecting against manipulation of any data or any algorithm used within the machine learning lifecycle, the confidentiality of sensitive data and intellectual property contained in it must also be protected, as the training data could e.g., reveal the inner workings of a component. Even the AI application itself or its underlying data about the relevance of specific training parameters might represent intellectual property in this respect.
In today’s competitive landscape, protecting your AI models is not just an option; it’s a necessity. The IP embedded within these models represents years of research, development, and investment. Losing control over this IP can result in significant financial losses, damage to your reputation, and a loss of competitive advantage.
Wibu-Systems’ CodeMeter family provides a comprehensive suite of tools to protect the IP in the finished AI models. From encryption and licensing to secure deployment and enforcement, our solutions are designed to be both powerful and flexible, allowing seamless integration into your existing workflows.
For example, CodeMeter Protection Suite offers several tools to safeguard both executables and data involved in AI and ML applications. Executables are protected from tampering or reverse engineering well beyond the traditional “security-by-obscurity” mechanisms. Executables or sensitive functions are encrypted using established cryptographic algorithms. In addition, cryptographic methods are utilized to protect the integrity of software and data. Functions and data are decrypted at runtime. Sensitive parts of the code can even be decrypted and executed, and key materials can be securely transferred and stored in secure hardware. This not only keeps the key material secret, but it also prevents their manipulation.
Due to the availability of open-source frameworks, as well as the popularity of the language, AI applications are often written in Python. AxProtector Python protects both the framework code used for training and the data used in the machine learning lifecycle, from manipulation, theft of intellectual property, and unauthorized use. If you would like to know more about protecting Python applications, watch our recorded Webinar, Protecting Python applications the simpler way.
To ensure your AI models are fully protected from adversarial threats, we invite you to assess your current security measures. Take a moment to fill out our brief form and evaluate if safeguarding your AI is a priority for your organization. Start here.
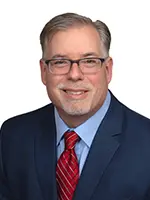
Contributor
Terry Gaul
Vice President Sales USA
Terry Gaul is a sales and business development professional with extensive experience in the software and technology sectors. He has been involved with software protection and licensing technologies for more than 20 years and currently serves as Vice President of Sales at Wibu-Systems USA. When he is not helping customers with software licensing, Terry typically can be found coaching his daughters' soccer teams or camping with his family on the Maine coast.